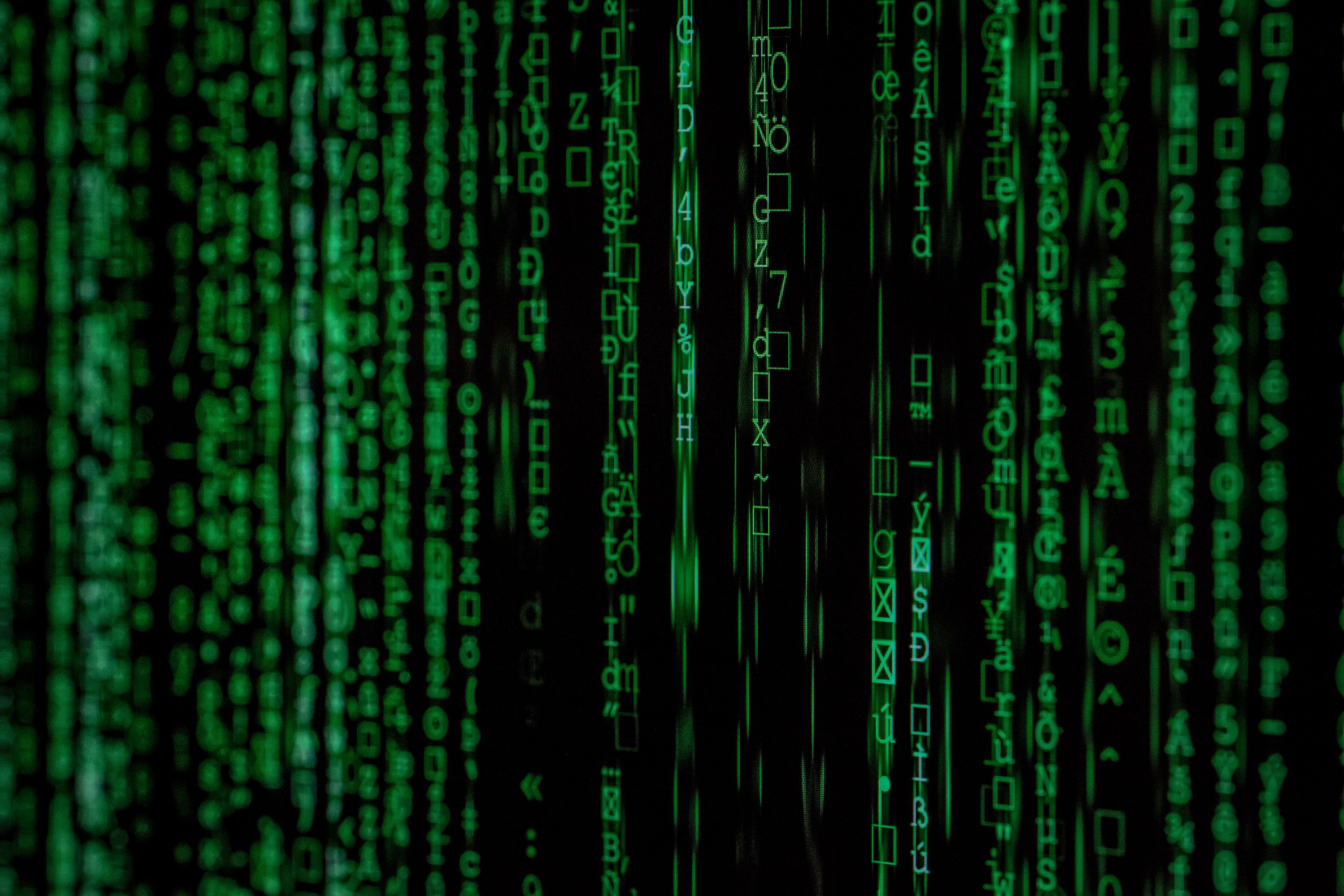
Hello Datascapers! I'm bringing this feature to you from Washington D.C., where I'm currently at a conference that is exploring public access to research data - an incredibly important topic of discussion (learn more here: https://www.aplu.org/projects-and-initiatives/research-science-and-technology/public-access/)! I've been working on this feature in the mornings at Philz Coffee in Dupont Circle, where they have some amazing green tea with fresh mint in it. This coffee shop is also right across the street from a venue where I saw the Descendents a few years ago, so that brought back some great memories of punk music and older punk music fans in suits and cardigans checking out the show after work.
Image Description: Divyansh smiling in front of a town center.
I am beyond excited this week to feature Divyansh Kaushik, a Ph.D. student in the Language Technologies Institute in the School of Computer Science here at CMU, who is doing some fascinating research that ultimately explores the question: how do we build machines that understand natural language? Specifically, he unpacks this question by trying to understand the underlying semantic concepts engrained in natural language text, with applications in various tasks such as question answering and identifying textual entailment. Divyansh shared two papers with me that highlight some of the unique work he's taking part in around these topics, and, as someone who is not particularly familiar with language technologies, it was an amazing learning experience for me!
Before I begin sharing with you the awesome ways these papers highlight how data are used in language technologies, let's do a brief introduction/refresher on machine learning: a method of data analysis where algorithms 'learn' from data, finding patterns and ultimately making decisions with minimal human intervention. This can be particularly useful for situations needing automation or prediction!
As shown in this graphic, the 'ingredients' which are fed into the machine learning model are data about a certain topic (in machine learning, these are often referred to as 'training datasets' for these models); in this case, data on eating habits, exercise, and healthcare. The algorithm then works to find patterns in the data, and develop predictive patterns to inform the strategies shown on the right side of the graphic, including the management of metabolic diseases based on data found in the training datasets.
I've been decently familiar with machine learning for a few years from my time working as a research associate at Oak Ridge National Laboratory in Tennessee, but the summary I just provided was the extent of my knowledge of machine learning, at least until Divyansh came along! As noted before, he shared two fascinating papers with me where he, and his coauthors, explore topics in machine learning as they apply to natural language processing, a subfield of study within artificial intelligence (AI) that explores how to enable computers to interpret and manipulate human language. This is why I love writing for Tartan Datascapes: not only do I get to meet all these awesome researchers like Divyansh on campus, but I also get to learn from their work!
The first paper is titled 'Learning The Difference That Makes A Difference With Counterfactually-Augmented Data' and can be found freely and openly (yay for open access!) at this link: https://openreview.net/forum?id=Sklgs0NFvr. With his advisors Dr. Eduard Hovy, Research Professor at the Language Technologies Institute, and Dr. Zachary C. Lipton, Assistant Professor of Operations Research and Machine Learning in the Tepper School of Business, Divyansh explored methods and resources for training models in natural language processing that are less sensitive to spurious patterns. These spurious patterns are an often-discussed topic in machine learning, and refer to a connection between two variables in the training datasets that incorrectly appear causal in that they have the appearance of directly affecting one another. This spurious correlation is often caused by a third factor that is not apparent when first observing the results of the model, sometimes called a confounding factor. The authors used a Human-in-the-Loop technique to approach this task - what does this mean? Remember earlier when I shared the definition of machine learning as a process for automation and prediction with little human intervention? Well, many researchers are realizing that leveraging human intelligence in machine learning can help produce a higher quality of results with less accuracy errors. While I'll let all you Datascapers read the paper for the full story (no spoilers!), I will say that Divyansh and his coauthors did find that this human intervention with the training data makes a difference in the quality of the results! Another thing that made my RDM Consultant heart happy is that the data are publically available at this link: https://github.com/dkaushik96/counterfactually-augmented-data. Yay for GitHub! Yay for Open Data!
The second paper is titled 'How Much Reading Does Reading Comprehension Require? A Critical Investigation of Popular Benchmarks', and can also be found open access at this link: https://www.aclweb.org/anthology/D18-1546/. Again with his advisor Dr. Lipton, Divyansh explored reading comprehension datasets used in machine learning, where the model should ideally extract appropriate answers to questions and passages of text. Many established datasets exist in the area of reading comprehension research, and the authors expressed new techniques and principles for evaluating how these datasets may be used more effectively, including a close inspection of datasets created programmatically. This hits at a really important point in research data management! It's important to take the time to ensure data is clean, organized, and free of any errors which can corrupt the results of the analysis. Again, I don't want to spoil the paper so I'll let all you readers go and download it, but believe me when I say that this is some fascinating stuff!
Data management is not always a one-size-fits-all approach; while there are general recommended practices for data documentation, filenaming, data formatting, storage, etc., we often need to tailor our data management to the unique needs of our data. Divyansh uses this same approach! When thinking about how to implement data management for his projects, he considers what kind of data he is working with, as well as the file sizes. This is an excellent way to strategically approach data management, and one of the reasons why I try to minimize my use of the phrase 'best practices' as they relate to research data management. How does Divyansh use this project-by-project approach to assess good data management practices? For the 'Learning The Difference That Makes A Difference With Counterfactually-Augmented Data' paper, he and his coauthors made the data publicly accessible on GitHub because of the (relatively) smaller file size. However, Divyansh notes that if it were to be a bigger dataset (in gigabytes order of magnitude), then they would have uploaded the data on their website and provided a script on GitHub to download it and work with it. For his own personal data management, he maintains a git repository on AWS so he can access his data anywhere, with version control.
Divyansh is a great example of the folks we have on campus engaging with fascinating projects that approach the use of data in innovative and important ways! I was lucky to get to ask Divyansh about his potential career goals, and he noted that he would like to do something that allows him to make the best use of both his skills in Machine Learning/Natural Language Processing, as well as in science policy, be it in academia or industry or for a non-profit in the policy sector. In the immediate future, he's going to be interning at Facebook AI Research in Summer 2020!
What are three takeaways from this researcher highlight?
1. Data management is not a one-size-fits-all approach, and oftentimes we need to tailor our RDM practices (filenaming, metadata, storage, etc.) to the unique needs of our data. Approach "best practices" with a critical lens, ensuring that they actually fit the needs of your data!
2. The quality of the training datasets in machine learning influences the quality of the resulting predictions and automations - taking the time to understand potential issues in the training datasets is worthwhile!
3. Version control is incredibly important when working with any kind of data, especially with large datasets. Platforms like GitHub are great for providing this version control, as it can be hard to remember each and every change we make to our data throughout a project.
Important Happenings in Research Data Management at CMU Libraries:
We have a great lineup of workshops coming up at CMU Libraries (click here to see our full list of workshops for the semester), many of which can help you learn new tips and tricks for data collection, analysis, and management. Here's a few that have a particular Tartan Datascapes-flavor:
dSHARP Gerrymandering Series: R Text Analysis, Monday, February 24th from 12:00 pm - 1:00 pm in the Sorrells Library Den (click here to register!)
Reproducible Data Visualization in Jupyter Notebooks, Thursday, February 27th from 12:30 pm - 2:30 pm in the Sorrells Library Den (click here to register!)
Cleaning Messy Data with OpenRefine, Thursday, March 5th from 12:30 pm - 2:00 pm in the Sorrells Library Den (click here to register!).
Data Management for STEM (taught by yours truly!), Monday, March 9th from 6:00 pm - 7:00 pm in the Sorrells Library Den (click here to register!)
And of course, please email me at hgunderm@andrew.cmu.edu if you'd like some help on your journey as a researcher/scholar/awesome human being here at CMU. Remember, we all use data, regardless of our discipline. If you think something might be data, you are likely correct and I can help you develop good habits for managing it! If you'd like to have your research data featured on Tartan Datascapes, please fill out this Google Form to get in touch!